TIPS #16: How can companies stay secure in the age of generative AI-powered innovation?
Shane Shook
May 31, 2024
- Blog Post
- TIPS
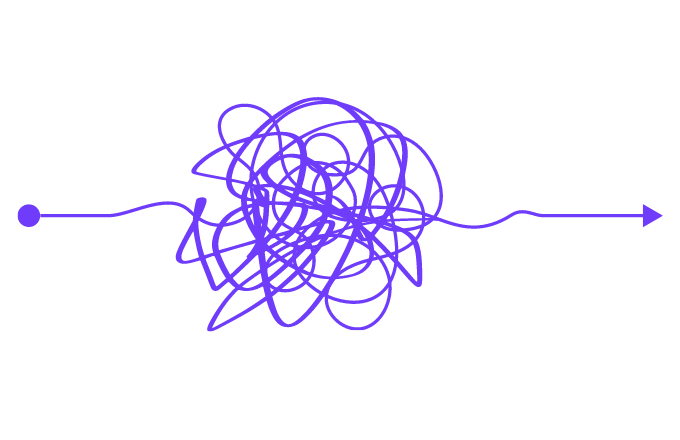
Issue: “The Messy Middle” – As generative AI (genAI) is rapidly adopted across business functions, many existing security control frameworks, tools, and practices do not adequately protect companies.
Generative AI (genAI) technologies have advanced significantly since late 2022 when ChatGPT became a household topic. Today, genAI is automating labor-intensive and repetitive work in areas including personal productivity (AI assistants), customer service (chatbots), software development (code generation), and cybersecurity operations (task automation in SOCs). According to the Cloud Security Alliance’s recent State of AI and Security Survey Report (commissioned by Google Cloud), over half of organizations plan to implement genAI solutions in 2024.
The addition of genAI solutions brings new technologies into company ecosystems not always set up for seamless or secure integrations. Companies face the possibility of misconfigurations, and current security controls and processes around data, access, and attack surface management often aren’t up for the task of protecting new AI use cases.
Data security challenges
Data security capabilities like data loss prevention (DLP) often focus on identifying and blocking the movement of defined data types. However, genAI models synthesize data and produce outputs that may bypass DLP and other similar filters. In addition, companies that use third party genAI models may share data with the models (and therefore third parties) for model training- but existing data security controls often lack the granularity to track and secure these data flows.
Access control issues
In many companies, internal data is already overly accessible to users due to poor access management. GenAI exacerbates this problem by enabling easy discovery and retrieval of data.
GenAI models can also create new data leaks when they are trained with permissioned data (pushed into the model through fine tuning, retrieval-augmented generation (RAG)- combining a large language model with an external data source, or another similar mechanism) and users without access to the underlying data are given access to prompt the model or view its output. This effectively gives users access to the restricted data.
Some genAI model integrations may also skip identity and access management (IAM) checks and bypass access control lists altogether.
Attack surface complications
It’s common for operators to evolve GenAI use cases and re-train models in multiple settings, making it difficult for traditional tools to track and manage potential attack vectors across data sources, applications, and endpoints. In addition, companies may be exposed to third party attack surfaces when teams and employees use unvetted genAI applications that don’t meet security requirements.
Threats to (and from) genAI models
GenAI models can be vulnerable to prompt injection (malicious inputs) and data poisoning (intentional manipulation of training data), both of which undermine model behavior. Security tooling that isn’t built to protect genAI models can’t defend companies against these threats.
In addition, most genAI models aren’t intrinsically factual and may be prone to hallucinations (producing outputs that aren’t based in reality), particularly if the training data is limited or if the model algorithms lack sophistication.
Impact: Companies face increased risks of insider threats, AI model manipulation, data leakage and theft, operational disruptions, reputational harm, regulatory violations, and fines.
Misconfigurations, inadequate security controls, and hallucinations can bring serious consequences to companies leveraging genAI. Companies may lose visibility and the ability to secure data and access permissions. Their attack surfaces can expand and threat actors may find new and under-protected targets, including the genAI models and data they interact with. Hallucinating models can also introduce uncertainty alongside inaccuracies, diminishing reliability and trust.
Companies may face higher risks of insider threats, genAI model manipulations, and data leakage, misuse, and theft. Sensitive or confidential data (employee, customer, company, or otherwise) may be exposed to unauthorized internal users and/or external parties and threat actors. Depending on the incident, companies can experience impacts including operational disruptions (especially in cases of ransomware), reputational loss, response and recovery costs, and fines due to violations of privacy or data protection regulations.
Action: Secure genAI models and the applications, data, and permissions they leverage.
1) Securing Identity and Access Permissions
To secure user permissions, companies can limit genAI model access to users with access to the underlying data feeding the model. Alternatively, they can fine-tune on non-sensitive data and provide sensitive data to the model via permission-gated RAG. This ensures that when the user prompts the model, they receive outputs based exclusively upon data they have permission to access.
In addition, a company’s broader access permissions environment and controls need to be clean and secure to enable successful genAI integrations. SPHERE remediates identity issues and protects critical data at scale so companies can reduce risk and accelerate innovation.
2) Mitigating Data Security Risks
Companies need visibility and control over their data systems and data flows, including the data genAI solutions interface with and rely upon. Symmetry Systems’ Data Security Posture Management (DSPM) platform visualizes and mitigates data security risks including data leakage threats posed by genAI integrations like Microsoft Copilot.
3) Protecting GenAI Applications and Dynamic Attack Surfaces
GenAI applications contribute to dynamic attack surfaces and require a proactive application security posture. Bishop Fox leverages offensive security expertise, penetration testing, and security assessments to protect complex genAI and machine learning ecosystems against threats.
4) Addressing SaaS Sprawl and Shadow IT (AI)
Companies need strong visibility and governance to guide employees to approved genAI solutions and build adequate data protections and security guardrails. Nudge Security’s patented discovery capability helps companies meet the deluge of GenAI and SaaS applications, reducing the risk of data breaches and privacy violations while enabling compliance via automated, user-friendly experiences.
5) Protecting against GenAI Hallucinations
Companies need visibility, controls, and guardrails to protect against hallucinations and other forms of unintended model behavior. Solutions may include:
- Developing more robust and diverse training data to reduce the likelihood of hallucinations
- Integrating human expertise to review model outputs (human in the loop)
- Implementing guardrails to narrow model outputs (based on security, safety, ethical, and other concerns)
- Using or developing explainability technology to understand model reasoning
- Investing in hallucination insurance to mitigate associated financial impacts