Special: Harnessing the Power of Generative AI in Cybersecurity
Alberto Yépez
July 18, 2023
- Blog Post
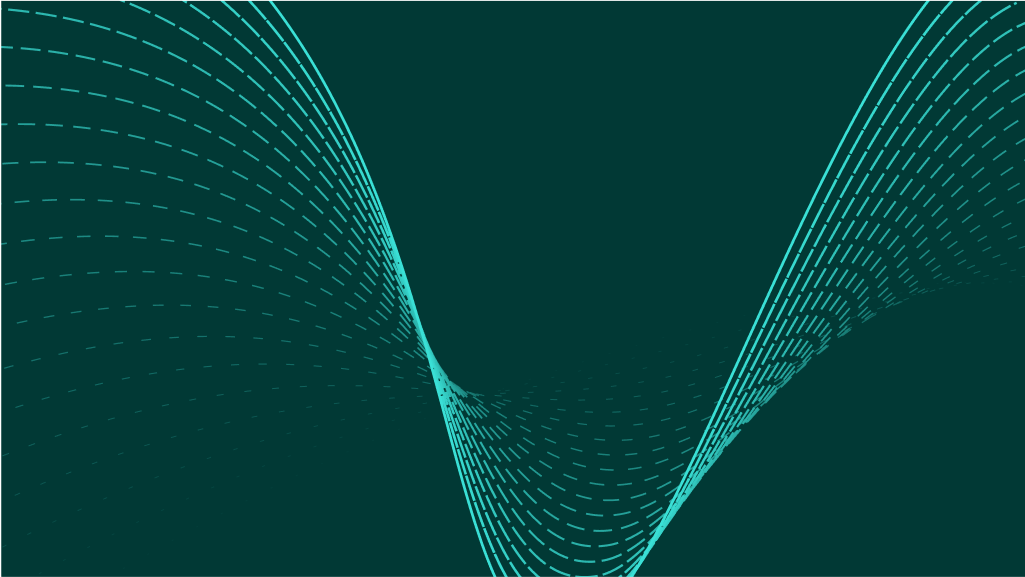
Over the past decade, BGV and Forgepoint have been investing in innovative AI and Cybersecurity startups. Alberto Yépez (Managing Director at Forgepoint Capital) and Anik Bose (Managing Partner at BGV and Founder of the Ethical AI Governance Group (EAIGG)) share their perspectives on how cybersecurity innovation will be impacted by Generative AI. Their joint theses is that Generative AI will enhance the capabilities of malevolent actors, increase the need for guardrails, with innovation advantage going to the incumbents in the near term and to startups for the longer term.
Artificial Intelligence is currently experiencing its “Netscape” moment, propelled by the advent of potent Generative AI models such as Chat GPT. Research conducted by McKinsey estimates that generative AI could contribute an equivalent of $2.6 trillion to $4.4 trillion annually to the global economy. (To put this into perspective, the United Kingdom’s total GDP in 2021 was approximately $3.1 trillion.) According to their analysis, about 75% of the potential value generative AI use cases could deliver is concentrated in four areas: customer operations, marketing and sales, software engineering, and R&D across industries. Unsurprisingly, AI is dominating conversations across the cyber world as businesses rapidly adopt and develop AI-based technologies- and/or react to their sudden rise and accessibility. So what are the implications on AI and Cybersecurity?
AI and Generative AI: Context and Definitions
Let’s begin with our context. AI is hardly new despite the intense hype cycle we find ourselves within. AI was first defined as an academic discipline in the mid-1950’s and has since gone through its own boom and busts – periods of intense interest (and funding) followed by “AI winters” and so on. Before the advent of Generative AI, our understanding of AI’s impact on cybersecurity was twofold. First, we recognized the application of AI for protection and detection, either as part of new solutions or as a means to bolster more conventional countermeasures. Second, we acknowledged the necessity to secure AI itself- both as a protective technology and as a tool used by threat actors to develop new attack vectors. Use cases varied from Transaction Fraud Detection, Botnet detection, File-based Malware detection, Network risk assessment, Vulnerability remediation, user authentication, endpoint protection (XDR), and spam filtering.
Today, with the release of several Generative AI platforms, we anticipate the Cybersecurity sector to be profoundly impacted in additional ways including:
- Amplifying the capabilities of malevolent actors through attack vectors such as evasion, extraction, and enumeration attacks.
- Bridging the cyber skills gap with powerful AI assistants, to boost the productivity of enterprise cyber teams. These include those launched by incumbents like Crowdstrike and Microsoft.
- Elevating compliance guardrails around data privacy and output data verification to ensure responsible AI deployment.
Before delving deeper, it’s essential to clarify a few key definitions:
- AGI (Artificial General Intelligence): AGI refers to highly autonomous systems that can outperform humans at most economically valuable work. AGI encompasses general intelligence and is capable of understanding, learning, and applying knowledge across a wide range of tasks. The goal is to replicate human-level intelligence, with the potential to exhibit self-awareness and consciousness. Our hypothesis is that Threat Intelligence Platforms (TIP) will shift towards GPT-like chats as a more effective information source for users, either as auto prompts and API feeds based on detection Indicators of Compromise (IOCs), or interactive for R&D, similar to how Microsoft Copilot is used forapp development, Security, and M365, and GitHub Copilot is used for programming.
- GPT (Generative Pre-trained Transformer): GPT is a specific type of AI model developed by OpenAI (for clarity, the popular ChatGPT is an AI chatbot app powered by GPT, similar to how a Lenovo or Dell laptop might be powered by Intel). Models such as GPT-3 and GPT-4 are designed for language generation tasks. They are pre-trained on large volumes of text data and can generate human-like responses given a prompt. These models excel at tasks like natural language understanding, text completion, and language translation. Our hypothesis is that AGI will improve interpretive systems (SOAR and Anti-Fraud) as Large Language Models (LLMs) and Small Language Models (SLMs) are harnessed for their most suitable functions.
New Attack Vectors: Enhancing the Capabilities of Malevolent Actors
Generative AI is a double-edged sword. While it holds immense potential for improving cybersecurity defenses, it also amplifies the capabilities of malevolent actors. By exploiting the capabilities of sophisticated AI models, attackers can devise new attack vectors that traditional security measures may struggle to counter:
- Evasion Attacks: In evasion attacks, the adversary uses generative AI to create inputs that are designed to be misclassified by AI-based detection systems. For example, they could manipulate malware so it appears benign to the security system, thereby evading detection. Generative AI, with its ability to understand and generate data patterns, can significantly improve the success rate of these evasion attempts.
- Extraction Attacks: Extraction attacks refer to scenarios where an adversary trains a model to extract sensitive information from a system, leading to potential data breaches. The advent of Generative AI means that attackers can train models to mimic the behavior of legitimate users or systems, thus tricking security measures and gaining unauthorized access.
- Enumeration Attacks: Enumeration attacks involve using generative AI to discover system vulnerabilities. Hackers can automate the process of testing different attack vectors, rapidly identifying weak points in a system that they can then exploit.
- Influence Attacks on Classifiers: Influence campaigns have been demonstrated in social media and securities/commodities trading systems’ reliance on AI repeatedly over the past decade or more – including election cycle and quarantine era mis/disinformation as well as the manipulation of market pricing and performance news. As generative AI is used for more specific, yet broader contexts and concepts in organizational functions, those same techniques will be exercised to exploit the dependencies on knowledge offered to organizations and consumers.
- Poisoning Attacks on Data: One simple example is in Copilot and generative AI code samples that hallucinate functions or resources that hackers may take advantage of to create malicious resources that are subsequently called by that code. This vulnerability requires code validation and testing before production release, which is generally a common activity in modern CI/CD development. This means that even development systems can be compromised and offer back doors for more nefarious software supply chain compromises, especially cine those development systems are rarely subject to network isolation or security controls levied on production systems.
As Generative AI continues to evolve, we anticipate an increase in these types of sophisticated attacks. Therefore, it is imperative for both incumbent and startup entities in the cybersecurity sector to remain vigilant and proactive, developing countermeasures that anticipate these new forms of threats.
While this may seem daunting, we believe it is also an opportunity for cybersecurity innovation. The challenges posed by generative AI-powered cyberattacks necessitate novel solutions, opening new frontiers in the cyber defense sector. Our discussions with key industry players reveal a robust willingness and preparedness to address these concerns.
Broad Yet Precise: Generative AI’s Impact on Cybersecurity Innovation
Generative AI has significant potential to influence cybersecurity innovation, both in established companies (incumbents) and startups. Here’s how generative AI is shaping cybersecurity:
- Anomaly Detection and Analysis: Generative AI models, trained on substantial datasets of known malware and cyber threats, can identify patterns and generate new threat signatures. This aids real-time threat detection and analysis, empowering security systems to proactively identify and respond to emerging threats. Generative AI models are used to detect adversarial attacks, where bad actors attempt to manipulate or deceive AI systems.
- Security Testing and Vulnerability Assessment: Generative AI can automate security testing by generating and executing various attack scenarios to identify vulnerabilities in software, networks, or systems.
- Password and Credential Security: Startups are using generative AI to develop password and credential security solutions.
- Malware Generation and Defense: Generative AI can be employed to generate new malware samples for research purposes and to strengthen antivirus and anti-malware systems.
- Security Operations Automation: Generative AI models can automate routine security operations while augmenting SOC analyst productivity.
The Need for Guardrails: The Generative AI Accuracy Problem
Generative AI has its limitations- primarily around consistently providing accurate outputs. Therefore, what guardrails are needed to reduce risks and ensure success with broader adoption? Generative AI tools like ChatGPT can augment subject matter experts by automating repetitive tasks. However, they are unlikely to displace experts entirely in B2B use cases due to AI’s lack of domain-specific contextual knowledge and the need for trust and verification of underlying data sets. Broader adoption of Generative AI will stimulate an increased demand for authenticated, verifiable data, free of AI hallucinations. This appetite will spur advancements in data integrity and verification solutions, alongside a number of other ethical AI issues such as privacy, fairness, and governance innovations. Boards of Directors now more vocally demand the responsible use of AI to improve operational efficiency, customer satisfaction and innovation, while safeguarding customer, employee and supplier data and protecting intellectual property assets.
On Near-Term Innovation: Incumbents’ Edge
Incumbents carry the advantage of pre-existing infrastructure, high-compute resources, and access to substantial datasets. Consequently, we anticipate a surge of innovation from these entities in the near term. Industry stalwarts such as Crowdstrike, Palo Alto Networks, Microsoft, Google, IBM and Oracle are already harnessing Generative AI to bolster their security solutions. Here’s an exploration of their endeavors:
Crowdstrike:
- Threat Detection and Response: Crowdstrike employs generative AI to detect and respond to advanced threats in real-time. Their AI-integrated platform, Falcon, scrutinizes large amounts of data to discern patterns and threat indicators, enabling swift detection and response to cyber threats.
- Adversarial Attack Detection: Utilizing generative AI models, Crowdstrike can detect and counter adversarial attacks like fileless malware and ransomware. Their AI algorithms are capable of pinpointing suspicious behavior, anomalies, and threat indicators.
- AI-Driven Security Analytics: By leveraging generative AI, Crowdstrike enhances its security analytics capabilities, thereby enabling the identification of intricate attack patterns, threat prediction, and the generation of actionable insights for security teams.
Palo Alto Networks:
- Threat Intelligence and Automation: The company integrates generative AI into their security platform, Cortex XSOAR, automating threat intelligence and incident response processes. Their AI algorithms sift through extensive threat data, equipping security teams with actionable insights and automated playbooks for efficient threat response.
- Malware Analysis: Generative AI models power advanced malware analysis. This helps companies understand emerging threats, devise effective countermeasures, and fortify cybersecurity solutions.
- Behavioral Analytics: Generative AI aids in developing behavioral analytics models that learn standard user, device, and network behaviors to detect anomalies and potential security breaches.
- Security Policy Optimization: By using generative AI, Palo Alto Networks optimizes security policies through the analysis of network traffic patterns, user behavior, and threat intelligence data, dynamically adjusting security policies for robust protection against emerging threats.
Microsoft
- SOC Automation: MS’s Security Copilot is a large language AI model powered by OpenAI’s GPT-4, combined with a Microsoft security-specific model that incorporates what Microsoft describes as a growing set of security-specific skills informed by its global threat intelligence and vast signals volume. Security Copilot integrates with the Microsoft Security products portfolio, which means that it offers the most value to those with a significant investment in the Microsoft security portfolio.
- Human-in-the-Loop Augmentation – While Security Copilot calls upon its existing security skills to respond, it also learns new skills thanks to the learning system with which the security-specific model has been equipped. Users can save prompts into a “Promptbook,” a set of steps or automations that users have developed. This introduction is likely to be resonant and disruptive because of the human aspect that remains — and will remain — so vital to security operations. The ability of large language AI models to comb through vast amounts of information and present it conversationally addresses one of the primary use cases of automation in SecOps: gathering the context of incidents and events to help analysts triage and escalate those that pose a significant threat.
Google:
- Vulnerability and Malware Detection: Google announced the release of Cloud Security AI Workbench powered by a specialized “security” AI language model called Sec-PaLM. An offshoot of Google’s PaLMmodel, Sec-PaLM is “fine-tuned for security use cases,” Google says — incorporating security intelligence such as research on software vulnerabilities, malware, threat indicators and behavioral threat actor profiles.
- Threat Intelligence: Cloud Security AI Workbench also spans a range of new AI-powered tools, like Mandiant’s Threat Intelligence AI, which will leverage Sec-PaLM to find, summarize and act on security threats. VirusTotal, another Google property, will use Sec-PaLM to help subscribers analyze and explain the behavior of malicious scripts.
IBM:
- Threat Detection and Response: IBM’s QRadar Suite is a subscription-based (SaaS) offering that combines AI-enhanced versions of IBM’s existing threat detection and response solutions into a comprehensive global product. The new QRadar Suite goes beyond traditional security information and event management (SIEM) capabilities, aiming to provide a unified experience for security management. Its goal is to assist organizations in managing extended detection and response (EDR/XDR) capabilities, SIEM functionalities, and Security Orchestration Automation and Response (SOAR) in cybersecurity.
- Security Compliance: IBM’s approach to security and compliance in highly regulated industries, such as financial services, emphasizes the importance of continuous compliance within a cloud environment. By integrating the Security and Compliance Center, organizations can minimize the risks associated with historically challenging and manual compliance processes. The solution enables the integration of daily, automatic compliance checks into the development lifecycle, ensuring adherence to industry standards and protecting customer and application data.
Oracle, SAP, Salesforce and other enterprise application providers are beginning to provide comprehensive AI service portfolios integrating their cloud applications and their existing AI infrastructure with state-of-the-art generative innovations. Their unique approach and differentiation means their customers will have complete control and ownership of their own data inside their “wall gardens” to derive insights and avoid data loss and contamination.
The incumbents not only have the company and customer install base and diverse platform to develop, test, and secure the safe and productive use of Generative AI / AI in general – but also having their own first party security products (Google’s Mandiant and Microsoft Security/Sentinel along with IBM’s Q Labs and Resilient acquisitions) that are using generative AI to power automated threat intel and security…while needing to retain human in the loop decision-making throughout the SDLC (and modern SOCs).
Longer Term Innovation: Advantage Startups
Startups offer innovative, agile solutions in the realm of generative AI for cybersecurity. However, the investment climate for generative AI-driven cyber solutions is still nascent, given the limited number of attacks witnessed to date involving the AI attack surface.
The pivotal role of data cannot be overstated. For startups to flourish, they must leverage open-source LLMs while enriching data with proprietary information. We anticipate that synthetic data innovation and Robotic Process Automation (RPA) will play crucial roles, especially in regulated sectors like financial services and healthcare that have unique data privacy requirements. However, synthetic data is not expected to significantly influence decision support automation, such as privileged access management.
Another key area for startup innovation exists around Verification and Testing, driven by mounting enterprise demand to harness Large Language Models (LLMs). Other noteworthy areas of opportunity include Explainability, ModelOps, Data Privacy for Generative AI applications, Adversarial AI/Data Poisoning, Autonomous Security Operations Centers (SOCs), Differential Data Privacy, and Fraud Detection.
Capital efficient startups will need to utilize existing infrastructure (foundational models) and concentrate on applications that add value through Single Language Models (SLM) via contextual data enrichment. Acquiring proprietary datasets may also be a strategic move for startups aiming to establish a competitive edge.
Furthermore, we posit that the compliance and regulatory environment shaped by the EU Act will direct startup innovation toward responsible AI and Governance, Risk Management, and Compliance (GRC). Notably, the founder DNA in this space will require a unique blend of cybersecurity domain expertise paired with generative AI technical prowess.
In Conclusion
We anticipate strong innovation at the intersection of Cybersecurity and Generative AI, fueled by incumbents in the near term and startups in the long term. Automating repetitive tasks with Security Co-pilots will go a long way towards addressing the cyber skills gap, while newfound protection and defense capabilities enabled by Generative AI will help secure large enterprise datasets and enable more effective identity orchestration to prevent breaches amid expanding attack surfaces. Morgan Stanley predicts that Cybersecurity is ripe for AI automation representing a $30Bn market opportunity. The bar on compliance guardrails will be raised in this space given the ethical concerns around the accuracy of Generative AI outputs (hallucinations), increasing the need for human-in-the-loop, regulations and raising the stakes to build an “ethics stack” to complement and safeguard the explosive AI technology stack. Finally, enterprise CTA’s (committees of technology and architecture) will increasingly need to embrace responsible application of Generative AI to succeed and compete.
Board of Directors will play an important role to demand good governance and the use of responsible AI, while protecting the key information assets of every business.
For those of you interested in joining us, we are co-hosting our inaugural Cyber AI Summit in San Francisco this fall at the NASDAQ Entrepreneurial Center. More details will follow shortly. Sign up for our newsletter and follow Forgepoint on LinkedIn for details.